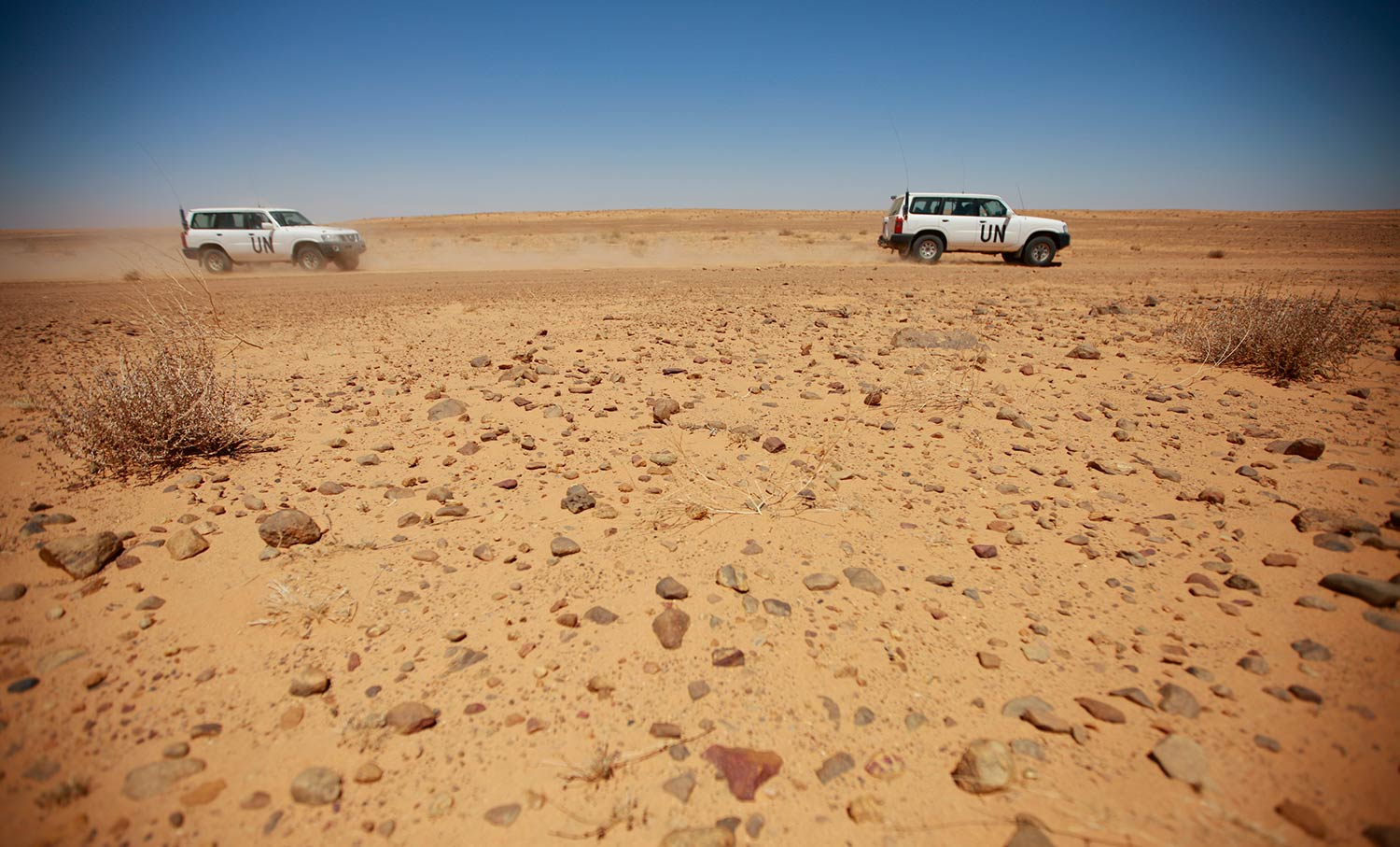
Accuracy of Our Forecasting Model
When presented with the Early Warning Project’s statistical forecasting model, users often ask how accurate they can expect the model to be. This page attempts to answer that question by showing how accurate the model has been historically using a visual summary and multiple ways of measuring accuracy.
Below, we explore the accuracy of the statistical forecasting model.
Photo above: Team leader Igor Buryan of Russia and colleagues Nestor Henriquez of El Salvador, Amara Kaba of Guinea, and Antonio Achille of Italy, members of the UN Mission for the Referendum in Western Sahara (MINURSO)'s Military Liaison Office, conduct a ceasefire monitoring patrol. June 15, 2010. Oum Dreyga, Western Sahara. UN Photo/Martine Perret.
How We Measure the Accuracy of Our Forecasts
Because onsets are relatively rare – with just one percent of countries seeing a new mass killing in any given year – describing accuracy is actually more challenging than one may expect. A model forecasting no new mass killings every year would have an accuracy of about 99% – an impressive performance but not useful at all to those hoping to identify high risk countries and prevent mass atrocities.
In our evaluation, we explore four ways of measuring the accuracy of our model’s forecasts:
- how much higher than average were risk scores in the years immediately preceding the start of mass killings,
- what proportion of mass killing onsets were captured by the Top-30 list produced each year,
- how often the model correctly forecasted mass killing that do occur, i.e. sensitivity,
- how often the model incorrectly forecasted mass killing when none occurs, i.e. precision
The analysis shows that while the statistical model performs well on three of these four metrics, its estimates are imprecise. The distinction between a new onset of mass killing and the continuation of an existing mass killing is important here. Because our project seeks to shine light on potential conflicts that are not already receiving attention, we evaluate our model only on its ability to identify the onset of new mass killings. Our accuracy depends on getting the timing exactly right. Forecasting a mass killing onset a year early counts against our model’s performance. In considering the model’s performance, this objective must be kept in mind. The analysis finds that:
- the average risk for years with mass killings (avg = 8% risk) is nearly three times higher than the average risk for years with no mass killings (avg = 3% risk)
- about two of every three (64%) of mass killings onsets since 1994 were captured by our Top-30 list in the two years prior to onset
- 71% of mass killing onsets since 1994 occurred in countries whose risk scores were 4% or higher in the year or two prior to onset
- if we classify countries with risk scores of 4% or higher as “high risk” countries, the model generates twenty “false positives” for every mass killing onset
This lack of precision is to be expected since mass killings are statistically rare. This is part of why we consider the Statistical Risk Assessment to be a starting point for further analysis and encourage users to consider a multi-method approach to early warning and risk assessment.
Generating Historical Forecasts
The first step towards evaluating model performance is to generate historical forecasts for all countries evaluated by the model. The Early Warning Project produced its first forecast in 2015 and made significant updates to the statistical model in 2017. As certain data became unavailable, two risk factors had to be removed from the model in 2020. The following analysis evaluates the model that the project used in 2020, applying it retroactively to previous years. We start this retrospective analysis in 1994, many years before the project started generating forecasts. We do this because there have only been three new onsets since the project began in 2015 (Ethiopia in 2015, Burma/Myanmar in 2016, and the Philippines in 2016), which is not enough data to speak systematically to the model’s performance. That said, the model forecasted two of these three onsets in its Top-30 List, which is roughly in line with our overall assessment of the model’s accuracy.
Model Methodology
For each year from 1945 until 2020, we have collected information on about 30 potential risk factors in about 160 countries. While the exact number of countries varies by year, the project includes all internationally recognized countries with populations of more than 500,000. We use these data to train a statistical model to forecast the onset of a new mass killing within the same country over the next two years. Specifically, we use a logistic regression, which is appropriate for binary outcomes (onset or not), and apply elastic net regularization, which identifies a subset of these variables with the most predictive power. For more details on this model, see the methodology section of our website. Some of the factors with the strongest predictive power are a history of mass killing, large population size, high infant mortality, and ethnic fractionalization.
The forecasts for the 2020-2021 window, and the relevant variables, can be found in our most recent report. Each year, we forecast the risk of new mass killing onsets during the next two years. Looking ahead two years allows us enough time to finalize and collect the necessary data and, we hope, gives policy makers enough time to respond to emerging crises.
Accuracy Assessment Methodology
In assessing the model’s accuracy, we apply this process to historical data starting in 1994 and generate forecasts as if the Early Warning Project had been active at that time. We choose 1994 as a cutoff because the instability associated with the end of the Cold War saw an unusual rise in the onset of mass killings. Starting the analysis after that global shift keeps conditions relatively stable and provides a reasonable point of comparison for how the model might perform in the future. This process generates 4,169 country-year risk estimates. We observe 33 mass killing onsets in the same period. We were unable to generate risk scores for 150 (3.5%) of these observations because our data sources did not have necessary information on all risk factors. For our most recent forecasts, data are consistently available and we are able to produce forecasts for every country within our scope.
Visualizing Highest Risk and Confirmed Mass Killings
The figure below shows the twenty-nine countries that either experienced a mass killing since 1994 or were estimated to have a 25% chance or greater of seeing a new mass killing onset. For each year, we averaged the forecasts made in the previous two years. Lower risk scores are shown in blue while higher risk scores are shown in red. White squares indicate that the country did not yet exist or that missing data prevented the model from generating a score. The last column of the figure is the most recent forecast. Black dots indicate the onset of mass killing and allow us to visualize how the model is performing. Many of these onsets (55%) occurred in the midst of mass killings by or against other groups, indicated by grey x-s. For example, the first row of the figure shows the onset of mass killing in Afghanistan in 1996 by the Taliban during efforts to crush domestic political opposition. These Taliban-initiated mass killings followed mass killings orchestrated by government forces attempting to defeat the Taliban.
Long High-Risk Periods
While not a formal metric of accuracy, the figure allows us to intuitively explore the model’s successes and failures. Many mass killing onsets occurred during periods of heightened risk, indicated by yellow, orange, and red tiles. For example, the risk of mass killing breaking out in the Democratic Republic of Congo during 1996 and 1998 was forecasted to be about 15% based on the forecasts made in the two prior years. The figure shows that the model generally identifies periods of about five years during which the risk of onset is unusually high. One likely reason is that the kinds of risk factors that can be collected across a larger number of countries for a long period of time tend to reflect enabling conditions for mass killing, which are generally slow to change.
False Positives
The model also identities time periods during which the risk of mass killing was high but no mass killings began. For example, the model estimated that the risk of mass killing in Sierra Leone remained above 10% from 1994 until 2003, and reached as high as 25%, but no new mass killings occurred. Whether these should be considered faulty warnings is difficult to evaluate. At any point in time, the model indicated that the most likely outcome was that no mass killing would occur, so this outcome is still consistent with the statistical forecast.
Evaluating Model Performance
Next, we introduce the metrics we use to judge the model’s performance. Deciding on the right metrics is important because some measures of model performance can be misleading. The most common metric to apply is accuracy, the number of correct classifications divided by the total classifications. The problem with this metric is that mass killings are extremely rare and a model that never forecasts any new onsets would be right about 99% of the time. Such a model would be of little help to policymakers trying to decide how to allocate resources and attention across potential conflict areas. Instead, we rely on four alternative metrics, drawing on scholarly literature about statistical forecasting of rare events:
- how much higher than average were risk scores in the years immediately preceding the start of mass killings,
- what proportion of mass killing onsets were captured by the Top-30 list produced each year,
- how often the model correctly forecasted mass killing that do occur, i.e. sensitivity,
- how often the model incorrectly forecasted mass killing when none occurs, i.e. precision
Metric 1. Average Risk Scores
First, we determine whether the model gave observations – i.e., country-years – that experienced mass killing a higher risk score than observations without mass killing. Pooling all of our data since 1994, we find that the average risk for country-years that did experience a mass killing within 2 years was 8% while the average risk for country-years without a mass killing was only 3%. In other words, the average risk for countries that did experience mass killings was nearly three times that of countries that did not experience mass killings.
The figure below shows the distribution of risk scores for countries that did not experience a mass killing and countries that did experience a mass killing.
Metric 2. Top-30 Coverage
Second, we assess what proportion of new mass killings were captured by our Top-30 list in any given year. The Top-30 list is one of the products produced by the Early Warning Project to draw attention to countries at high risk. For the model to perform well, we would want this list to capture most mass killing onsets. The figure below shows the number of mass killings that were included in the Top-30 rankings two years prior to occurring and the number that were not. Since 1994, the Top-30 list captured 21 of 33, or about 64%, of mass killings onsets. The missed onsets are distributed evenly over time and space although in three of the twelve missed cases, data limitations prevented the statistical model from making a forecast (Somalia in 2007, Syria in 2011, and Syria in 2012).
Metric 3. Sensitivity
Third, we measure the model’s sensitivity, that is, how often the model correctly forecasted mass killings that did occur. Because the model generates probabilities rather than yes/no assignments, there is no single threshold at which to evaluate the model’s sensitivity. Classifying countries in the Top-30 list as “high risk” is one way to draw this line but there are many other plausible thresholds. In fact, by changing this threshold and setting it very low (e.g. to classify all countries with more than 2% risk as high risk), we could make sure that our model captured all or most of the mass killings that did occur. By setting the threshold very low, however, we would also be making a lot of false positive forecasts and our model would not be very specific. The receiver operating characteristic (ROC) curve, shown in the left-side plot below, allows us to capture the model’s sensitivity, and the tradeoff with specificity, at every potential threshold.
For each threshold, we measure the model’s sensitivity (shown on the y axis) and compare it to the model’s specificity (shown on the x axis). Specificity measures how often the model incorrectly forecasts a mass killing when none occurred. Each point on the plot is a different threshold for classifying a risk score as forecasting a mass killing. For example, the highlighted point tells us the sensitivity and specificity when we classify all cases with a risk estimate of 4% or higher as instances of potential mass killings. We use this 4% benchmark to identify countries at high risk of mass killing because it tends to correspond with the Top-30 list. The figure shows that if we classify countries with risk scores of 4% or higher as “high risk”, we correctly identify 71% of mass killing onsets since 1994.
The ideal model has both high sensitivity and high specificity. We can determine a model’s performance by comparing these proportions against a naive model which classified cases randomly. This naive model makes false forecasts at the same rate as true forecasts, shown by the red line. In contrast, our model makes more true forecasts than it does false forecasts, so its green line lies above the naive model. If we calculate the area of the graph that lies below this green line, we get the area under the receiver operating characteristic curve (AUROC) statistic, which measures model performance. The best performing models will be much higher than the naive model and have an AUROC of .9 or higher. Our model has an AUROC of .85, meaning that it performs reasonably well. To explain this same statistic another way, the AUROC tells us that if we randomly draw a historical incident of mass killing and compare its risk score to a randomly drawn non-mass killing, the mass killing would receive the higher risk score about 85% of the time.
Users might also be curious to know how the model’s performance holds up over time. Other projects have been critiqued for fitting historical data very well but producing less accurate forecasts when applied to current conditions. One of the advantages of our approach is that we retrain our model on the newest available data each year, allowing it to learn over time. This approach appears to yield benefits, as seen in the right-hand side of the plot, which shows how the AUROC has changed over time. We measure this statistic for every year from 1994 to the present, using a two-year bandwidth on either side to ensure that there are enough mass killing onsets to measure the model’s performance. The figure shows that our performance is steady over time and oscillates between a low of 75% and a high of 95%.
Metric 4. Precision
We explore the model’s ROC curve because it is a standard metric in predictive analytics. However, when the outcome is rare, as it is for mass killings, the tradeoff between sensitivity and specificity can be misleading because it is easy to correctly forecast no mass killing when none occurred. When the outcome is rare, precision is a more rigorous standard by which to evaluate a model. It measures the proportion of countries that were forecasted to experience mass killing that did have a new onset. A more precise model makes fewer false mass killing forecasts. A graph showing the precision and the sensitivity is called a precision-recall curve (PR curve), where recall is just another name for sensitivity. As with the ROC curve, we can determine a model’s performance by comparing the tradeoff between precision and sensitivity of our model against a naive model.
The PR curve, shown on the left-hand side of the figure, reveals that the model generates a large number of false positives for every correct forecast. The figure shows that there is no threshold at which the model produces more true positive classifications than it does false positive classifications. In short, there are more non-mass killings than there are mass killings no matter which threshold one chooses to define “high risk,” which is what we would expect based on the rarity of mass killings. In fact, if we choose a threshold that correctly identifies at least 80% of the instances of mass killings (i.e. recall/sensitivity of 80%), our precision would be less than 5%, meaning that more than 95% of our forecasts would be false positives. If we classify countries with risk scores of 4% or higher as “high risk” countries, the model also has a precision of about 5%, meaning that it generates about twenty false positives for every mass killing onset. Looking at the AUPR by year, shown on the right-hand side, this pattern is largely constant with a large number of false positives during every time period and reaching a maximum AUPR of .16.
This analysis was prepared by Vincent Bauer, Early Warning Project Data Consultant.